Data Quality Management in PIM: Best Practices for Ensuring Accuracy and Consistency
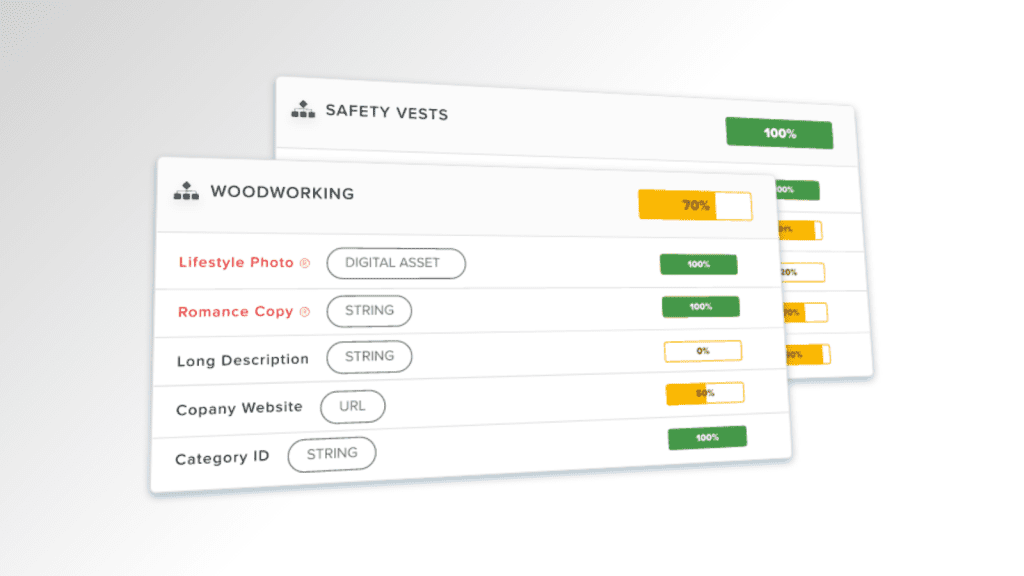
- Data quality management is critical for success in PIM.
- Best practices include defining standards, using PIM to monitor and visualize critical metrics, and regular reviews.
- Brands with sound data gain a competitive advantage in their industry.
In this Article
Online shoppers like it when they can make the right choice quickly. Research indicates that poor product information quality (PIQ) creates undesirable online shopping outcomes. This means shoppers will likely abandon the site for alternatives if they receive unhelpful and low-quality product information.
Shoppers find your product information of poor quality if:
- The information presented is inaccurate.
- Product descriptions provide insufficient details about features, benefits, and usage.
- Images are too small, blurry, or do not fit the context.
- Customer reviews are unavailable.
- The information is outdated.
- The information is inconsistent across different platforms or channels.
However, how can you tell that you’re serving poor PIQ? Many brands have systems to govern product information storage, management, and sharing. The beating heart of the system encompasses meticulously designed data quality management principles. They include early warning systems and processes that identify and rectify inconsistencies, ensuring product information remains reliable and trustworthy across all touchpoints.
Does this system sound familiar? If not, chances are high that you do not have an established means of knowing what kind of product information you serve to customers, and this post is all you need to take the crucial first step toward rectifying the situation.
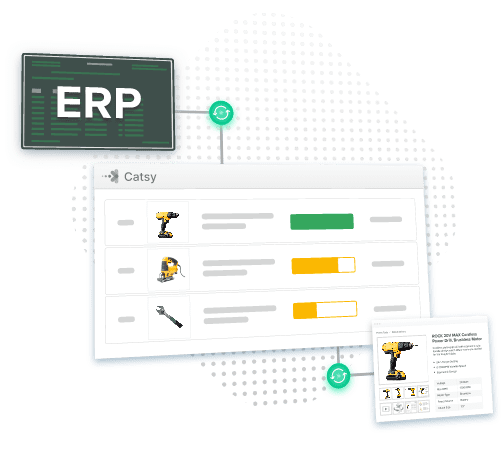
Understanding the Basics of PIM and Its Value for Industrial Brands
What is PIM?
Product Information Management (PIM) is both a system and a software tool designed to address the challenges of product data. As a system, it establishes a structured approach for centralizing product information from various sources (like spreadsheets, ERP systems, or supplier data). The PIM software acts as the core of this system, providing the tools for:
- Consolidation: Bringing together all product-related information into a centralized repository.
- Management: Organizing, enriching, and maintaining the accuracy of product data.
- Sharing: Distributing consistent, up-to-date product information across various sales and marketing channels.
In other words, PIM software is the foundation for brands to build a robust system for managing product information.
Benefits of PIM for Industrial Brands
- Managing complex data: Industrial products often have many details, including technical specifications and certifications. This makes data management unwieldy without a specialized tool like a PIM.
- Omnichannel consistency: PIM systems ensure that product information is accurate and up-to-date across all customer touchpoints – from your website and catalogs to industry-specific marketplaces.
- Faster time-to-market: Most robust PIM tools provide templates and automation features that expedite product data consolidation and enrichment. The result is that brands can respond quickly to market demands.
- Improved collaboration: PIM platforms allow several stakeholders (with appropriate authorization) to access and edit product details within the repository. This shared workspace promotes efficiency and collaboration throughout the product lifecycle.
- Enhanced customer experience: A PIM tool aims to provide accurate, detailed, and easily accessible product data. Once syndicated to channels, shoppers can use high-quality data to make informed decisions faster, increasing satisfaction and improving sentiment towards your online storefronts.
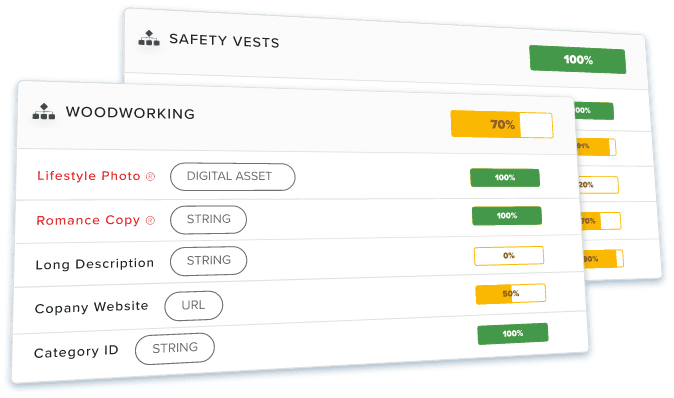
Definition and Importance of Data Quality Management
What is data quality management (DQM)?
Data quality management (DQM) refers to brands’ practices to maintain a high PIQ (product information quality). Typically, brands follow a set of practices throughout data handling, from acquisition to storage, enrichment, and distribution.
Technically, DQM ensures the accuracy, completeness, consistency, reliability, and timeliness of data within an organization. As part of product information management, DQM focuses on the quality of product information flowing through the system. It involves several critical processes, including:
- Profiling and analyzing: Evaluating your existing product data to identify errors, inconsistencies, missing information, and outdated elements.
- Establishing standards: Defining clear guidelines for data formats, permissible values, and mandatory fields.
- Cleansing and enrichment: Correcting errors, standardizing formats, filling in missing details, and enhancing product descriptions for optimal impact.
- Monitoring and governance: Implementing ongoing monitoring processes to track data quality metrics and establish procedures for addressing new quality issues.
Dimensions of data quality
Data quality isn’t a binary on/off switch. Instead, it is a spectrum reflecting the overall health and trustworthiness of product information flowing through your organization.
Imagine data quality as a fitness tracker for your brand’s product information. Like physical fitness has various dimensions (cardiovascular health, strength, flexibility), data quality encompasses several key aspects, including:
- Accuracy: Does the data accurately reflect reality? Think of a product specification listing the wrong voltage requirement for a power tool. Inaccurate data can lead to wasted resources, safety hazards, and frustrated customers.
- Completeness: Is all the necessary information present? An incomplete customer record missing a phone number can hinder communication and delay critical service calls.
- Consistency: Is the same information presented in the same way across all channels? Imagine encountering conflicting product descriptions on a website versus a printed brochure. Inconsistency confuses the end user and undermines trust in your brand.
- Timeliness: Is the data up-to-date and reflects the latest information? Relying on outdated product specifications can lead to safety risks or compatibility issues.
- Validity: Does the data adhere to predefined standards and expectations? For instance, a description formatted incorrectly or a product code that falls outside the designated range would be flagged as invalid. Validity ensures your data conforms to established criteria, further enhancing its reliability.
Note: The level of data quality required will vary depending on the specific context. For instance, a marketing list with a small percentage of duplicate names might be acceptable for a broad awareness campaign. However, if you're dealing with technical specifications or regulatory compliance, even a minor data error can have significant consequences, like hefty fines or reputational damage.
Impact of poor data quality on business operations
According to Gartner, organizations lose an average of $12.9 million annually because of poor data quality. However, the question is: how does this relationship play out?
- Inconsistent product information across channels: Imagine a customer who recently sampled your products in a digital catalog elsewhere, finding that the descriptions on your online store do not match. This inconsistency will confuse the shopper and undermine trust in your brand’s believability. Nothing is worse than inconsistency in business-to-business (B2B) – B2B buyers want precise details to avoid compatibility issues, wasted resources, and even safety hazards.
- Operational inefficiencies and increased costs: Poor PIQ creates a ripple effect of inefficiencies across departments. For instance, sales teams may struggle to provide accurate product information to potential buyers, leading to lost sales opportunities. On the other hand, the marketing efforts based on inaccurate customer data yield poor results. Additionally, technical support teams may face a backlog of inquiries due to inconsistencies in user manuals. This translates to wasted time, resources, and increased operational costs.
Features of PIM Software That Support Data Quality Management
PIM tools offer several built-in capabilities for maintaining the health of your product information. Some examples include:
Data validation and cleansing
Validation refers to the practices you undertake to ensure product information adheres to predefined standards. One of the most essential capabilities of robust PIM software is defining and automating data quality rules. The goal is to evaluate product information against specified criteria to determine suitability. This could include checking for completeness, conforming to standard formats, value ranges, taxonomy rules, etc.
For example, a power tools manufacturer can set up data entry rules that may include things like mandatory fields, character limits, or specific formatting requirements (e.g., dates must be entered as MM/DD/YYYY, “Product Name must not contain special characters,” or “Net Weight field must be a numeric value between 1-5000 lbs.” These rules proactively prevent errors from being introduced.
From there, data cleansing functions can standardize, parse, translate, or correct specific data issues. Let’s say the PIM tool highlights a consistency error across product descriptions for a particular product. You can use batch editing capabilities to correct these issues across hundreds of products and several channels at once.
Workflow management and approval processes
Defined workflows do an excellent job when it comes to reducing errors. This is possible because PIM tools allow you to set up custom workflows based on your team’s structure.
For example, the product management team would validate new or updated product data for an industrial HVAC system for specs accuracy, the technical documentation team for attribute completeness, and the localization team for translations – all before final approval. This ensures a reliable control point and segregation of duties.
The point is that workflows create accountability. Changes to product information (or new data entry) go through proper checks, closing any cracks that may let unapproved or inaccurate information slip through.
Version control and audit trails
The thing about PIM software that sets it apart from rudimentary tools like spreadsheets is the ability to track changes. With the latter, you’d have to maintain several copies of the same data for reference, which is hectic. On the contrary, PIM tools like Catsy store different versions of the same data so you can revert quickly when needed.
Since each user with editing rights must have authorization, PIM tools record every aspect of the action: what change was made? Who made it? When was it made?
For example, a brand can analyze who altered pricing data and when for a product line to investigate inconsistencies. Or they could “roll back” incorrect product descriptions to a prior approved version. This supports monitoring, remediation, and learning for continuous data quality improvement.
One can conclude that the version control aspect of PIM software builds transparency and accountability.
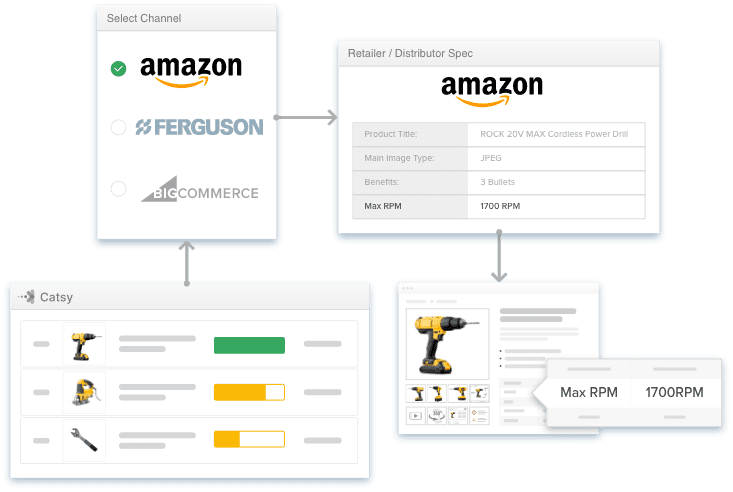
Best Practices for Data Quality Management in PIM
Establish context-based data quality standards for your product information.
Knowing what best suits your brand is critical to ensure product information quality (PIQ). It entails defining a “good enough” standard for your data. This first step is necessary because data quality is more than one-size-fits-all. Instead, it’s a balancing act between perfection and practicality. Most importantly, the standards should align with your business goals and customer needs.
The question then arises: how do you approach context-based data quality standards?
First, prioritize data fields. This step is critical because different details have different levels of importance in specific contexts. For example, you could categorize data fields as follows:
- Critical fields: This will include product names, SKUs, core technical specifications, and primary images. Here, accuracy is non-negotiable.
- Important fields: Think of detailed descriptions, secondary images, and supporting documents. A high standard is desirable, but some room for error might be acceptable.
- Nice-to-have fields: Often, this includes things like marketing taglines or cross-sell recommendations. Data quality here is a bonus, not an essential requirement.
Secondly, consider the use case of each data asset. For example:
- Customer-facing data: Information visible on your website or in catalogs must be meticulous to build trust and reduce errors in ordering.
- Internal data: Inventory levels or supplier codes used mainly for operations might tolerate a slightly lower quality threshold.
Lastly, set measurable targets. Instead of vague notions of “good,” define quality in specific terms, such as:
- “99% accuracy for critical fields”
- “No more than 5% missing information in important fields.”
For example, a heavy machinery manufacturer might deem a 5% error rate acceptable in its internal-use field for “estimated shipping weight.” However, they would likely have a near-zero tolerance for mistakes in the machine specifications visible to customers.
Defining the “good enough” data standards in PIM matters because clear, customized standards guide data cleansing and enrichment efforts. They create a shared understanding for your teams and prevent wasting time on perfectionism where it’s not strictly necessary.
Establish a data quality (DQ) standard across the organization
With the “good enough” definition established, the next step is to develop an organization-wide framework to guide DQM. This process is essential for data quality management to be truly effective – it builds cohesiveness across the entire organization.
How can you make this happen?
- Encourage cross-departmental collaboration: Data quality is everyone’s business! So, get input from all departments that interact with product information. They should offer valuable insights into the most critical data for their workflows and customer interactions.
- Implement a data governance framework: Develop clear guidelines for data entry, formatting, updates, and access permissions. Some key elements include:
- Standardized naming conventions (SKUs, file names, etc.)
- Requirements for mandatory fields and metadata
- Guidelines for data updates and versioning
- Assignment of data ownership and accountability for different categories of product information
A robust data quality framework enables brands to bake quality into data from creation and capture through transformation, storage, and distribution.
For example, a power tools manufacturer may incorporate data quality approvals into new product development processes, so no product data gets published without certification that it meets all quality standards first.
This unified data quality approach avoids duplicative and inconsistent efforts between divisions and provides much-needed transparency and accountability. However, global quality guidelines should still allow appropriate flexibility to handle the nuances of different data domains.
Design and implement DQ dashboards for monitoring critical data assets
Constant monitoring is the surest strategy for maintaining a desirable product information quality. Data quality isn’t a “set it and forget it” task.
Robust PIM tools like Catsy offer built-in dashboards and tools to help you monitor product information quality. These dashboards act as a command center, providing real-time visibility into data quality scores, metrics, trends, and issues across the organization.
The equivalent of readiness dashboards in Catsy PIM is a readiness reporting tool. This feature computes a data quality score for data objects based on customized rules. It then visualizes the score in the Data Objects editor, giving users an at-a-glance view of the data quality status. The feature also provides improvement suggestions according to the computed score, helping users identify areas for improvement and take corrective action.
Additionally, the readiness reporting feature allows organizations to add a precondition for computing data quality scores only for certain kinds of data objects. This enables them to monitor and improve the quality of specific data assets critical to business objectives.
Generally, the data quality dashboards allow you to identify and focus on tracking the right metrics. Some examples include:
- Data accuracy: How well do data values match the real-world entities they represent?
- Data integrity: Does your data conform to defined rules, data types, and business expectations?
- Data consistency: Is information formatted in a standard way, and are there no contradictions across sources?
- Data completeness: Do your records contain all required values and information?
- Number of empty values: How many missing fields or placeholders exist within your data?
- Data time-to-value: How quickly can you extract actionable insights from your product information?
Most importantly, configure your dashboard to send alerts when key metrics fall below acceptable levels, enabling proactive intervention.
Communicate the benefits of better DQ regularly to business departments
A data quality management framework can succeed only if sufficient buy-in exists. In other words, data quality initiatives are most effective when the entire organization understands and buys into their importance.
So, industrial brands should regularly communicate the tangible business benefits of maintaining high data quality standards across all departments. For example:
- Sales teams: Accurate data increases conversions and minimizes returns.
- Marketing teams: Reliable data fuels effective campaigns and reduces wasted ad spend.
- Customer Support teams: Complete information enables fast and accurate issue resolution.
Most importantly, encourage feedback from other departments to make this a two-way conversation. They might have unique insights into how data quality issues impact workflows and customer outcomes.
Review and update progress regularly to make timely corrections and checks
For emphasis, data quality management is not a “set it and forget it” task. Regular review and updating are required to keep the bar high. Some tactics for creating a cycle of continuous improvement include:
- Regular reviews: Track your data quality metrics over time. Are you seeing improvement? Are any specific problem areas persisting? This informs where you need to adjust your strategies.
- Adapt and refine: Based on your monitoring, update processes, adjust data quality rules, or implement new tools. Don’t be afraid to experiment and find what works best for your specific data and business requirements.
- Knowledge sharing mechanisms: Establish ways to share best practices across departments. This could include:
- Internal documentation or a knowledge base
- Regular training sessions or presentations
- Company-wide newsletters or announcements highlighting data quality wins
Conclusion
While Data Quality Management (DQM) might seem complex, the long-term benefits far outweigh the initial investment. This significance cannot be overstated, especially in the current B2B sphere, where data is the fuel driving operational efficiency and customer satisfaction. So, prioritizing data quality is not optional; it’s a strategic imperative.
Sticking to best practices like establishing data quality standards, leveraging PIM tools for monitoring, and fostering a culture of data ownership is a solid strategy for success. This approach can transform data quality from a burden to a strategic advantage.
Inaccurate or incomplete product information can lead to severe issues, like safety hazards, compatibility problems, and wasted resources. Data quality management (DQM) ensures your product information management (PIM) system provides reliable data for informed decision-making.
For industrial PIM systems, “good enough” data considers the criticality of the information. For example, a minor typo in a description might be acceptable, but an error in voltage rating for a power tool could be a safety risk and require stricter accuracy standards.
DQ dashboards act like a cockpit view for your product information health. They provide visual insights into data quality metrics, allowing you to proactively identify and address potential issues before they snowball.
Showcase the benefits! Regularly communicate how improved data quality translates to increased efficiency, reduced errors, and a stronger bottom line for everyone in the organization.
Absolutely not! DQM is an ongoing process. Regular reviews, sharing successful best practices, and adapting your strategies as needed are crucial for maintaining a culture of continuous improvement in data quality.